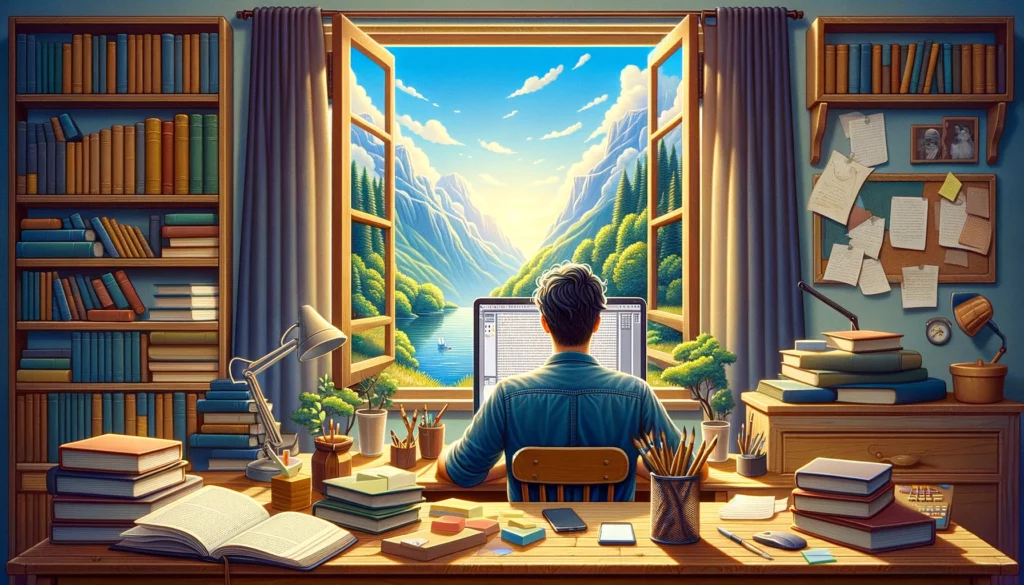
Learn the limits of Local LLM and never use them again unless you have no choice and find out you can do it then.
Introduction
In this blog post we are going to talk about local LLM and why it is nearly never the solution and you should not use it. This may sound hard but this is the point of view of someone who used most of those solution and push LLM powered application on production.
More precisely, you will learn in this post:
- What is a local LLM, what is self deployed LLM and LLM services
- The pros and cons of each strategy
- A specific focus on privacy and GDPR
- A cost analysis of each strategy
What is local LLM
A local Language Learning Model (LLM) refers to an LLM model that operates on a user’s own hardware or local infrastructure, rather than being hosted on cloud-based servers. This means it runs on either your computer, server or even your own datacenter (if you have deep pockets).
There are multiple framework to have local LLM (check GPT4All or Llama.cpp)and it is working fine. They are wonderful framework that allows to run local LLM, use them as a chat and do not even need GPU.
Pros
- There are multiple framework to have local LLM (check GPT4All or Llama.cpp)
- They are working fine and does not need GPU
- Tens of LLM available to use easily
- You have garenteed privacy of you data
Cons
- A LLM model is inherently very big (the smallest one are already +1GB) and needs a lot of compute power so you still need a pretty neat computer (don’t think you can launch this with your old laptop)
- The LLM that you will use will be either 7B (meaning that they have 7 billions parameters inside so very small for LLM) or quantized models (the parameters used are not as precise) so the LLM will be less relevant (in theory). Check out our post on LLM explained in 5 min.
- You can’t expect them to be as powerful as ChatGPT (GPT4). GPT4 runs (by the latest leak) with 128 A100 GPUs (one A100 has 80GB of GPU memory and cost +10 000$). That means the LLM will be dumber, will accept far less input text and give small sized answer (This is literally writed in the homepage of GPT4All)
- You will need to have the LLM launched all the while you need it which means more energy consumption and you will degrade your computer faster
- If you use for prototyping an app, for example with langchain (see our other posts for tutorial on how to use langchain), at some point you will want to make it available to other or use another some other place. You cannot have your laptop working 24/7. You will also need to handle all the errors, load balancing, monitoring and all the nice stuff when creating an application or a service by yourself.
Cloud hosted self deployed LLM
This is a very long naming for just saying you will use an LLM that is deployed on the Cloud (Amazon AWS, Google GCP, Microsoft Azure) and not on your local machine.
Pros
- You could deploy bigger models than locally (you could deploy a full Mixxtral 8x7B which is the current best on open sourced models with a total parameters of 56 billions parameters)
- You can create a full fledged LLM service by yourself and learn how all these big LLM provider works
- You can deploy your own LLM just for heavy batch processing and delete it after use. This will allow you to be super cost effective.
- The only limit is your knowledge and the depth of your pockets (it costs a lot)
- This is very good if you want to test a new shiny model for example
- Very good for privacy as you really master your LLM deployment and usage
- You can easily create application using langchain and use it like another LLM service
- Check out this link on how to deploy an LLM in 5 min in AWS and see by yourself how to do it
Cons
- You really need some skills and time to do that (I actually do that in my work and I guarantee that it is not easy at all and you needs some serious experience and a great team to pull it off)
- It costs a lot (a full Mixtral 8x7B would cost 4$ dollar per hour which means 40$ per day for barely 10 hours of usage)
- You need to handle all the nice stuff of an application but it is easier that local LLM as you have the cloud resources that makes all this easier
- You pay for the time the LLM is deployed so if it is deployed for a whole week even if you are only using it 2h a day, you will pay for the whole week
LLM Services
This is actually the well know LLM service (like OpenAI) that you can use in your everyday usage.
Pros
- Pay per use billing strategy where you pay only what you really use. More precisely you pay for the number of input tokens (size of your input) and the number of output tokens (size of the output response)
- There is no tedious task apart from creating an account on their system and create a token to be used in your application (simply put just some credentials to use in your app). You can literally have a working minimalist app in 5 min (see our post about that)
- All the tedious task of managing the LLM is taken care, you can concentrate on your use cases
- Very well integrated with the LLM frameworks (langchain and llamaindex)
- It is very cheap for prototyping (at the beginning)
- You can access multi modal models (models that accept image or can give images) which are inherently more complicated and more resources hungry
- You can access their proprietary models which are really powerful. For example GPT4 is a 1.8 trillions parameter models, if you compare it with a Mistral 7B, that is more than 25x bigger.
- Check out our post that list the LLM services and their pros/cons.
Cons
- You could have privacy or GDPR issues. Most of the well know LLM services have terms of use where they agree to not use your data when using their services but you should always check. Even more if it is free (then you are the product).
- It costs a lot when using in large scale if you do not have a good architecture. We will see a little more in the next part.
Privacy and GDPR
Privacy is an important part of the each use case, whether it is a small web app or a full fledged 1M+ users. In either case, if you have users, you need to be concerned about the privacy of their data.
In the same topic, GDPR (General Data Protection Regulation) is a set of European Union regulations designed to protect the privacy and personal data of individuals within the EU and the European Economic Area.
Basically, what is required is to:
- be transparent about what data you are using
- minimize the collected personal data
- Asks consents
- Give a way for an user to delete its account and the related data
So what is the link with LLM ? Well, then, depending on the service you are using, you could be giving all the data you are collecting to the service you are using. That is why you need to be careful about this.
And good thing, the biggest LLM providers (OpenAI, AWS, Azure, GCP, MistralAI, Anthropic) already write in their service offer that they will not use your data for their own scheme.
What’s more, if you are based in Europe and if you are using a big cloud provider (AWS, GCP, Azure) or MistralAI, you will be guaranteed that the LLM model that you will use as well as the data will stay in a data center in Europe.
Cost analysis of each strategy
Strategy | Development cost | Running at scale costs | Difficulty to prototype | |
Local LLM | 0$ (only the price of your laptop) | Not possible | Very difficult, you need to redo the application | |
Cloud hosted self deployment | At least 1$ per hour (when the model is deployed) | Pay for the size of the model deployed | Difficult because you need to literally create a working LLM service for yourself | |
LLM services | Pay per uses ( 0,1$ per million tokens) | Pay per uses BUT this can become very expensive | Just concentrate on the actual application developement |
As you can see, using an LLM service gives the best trade-off between running cost, development and difficulty to prototype.
The only way the Cloud hosted self deployment makes sens is if you have a very big bill on your LLM service and you have already implemented all the cost saving strategies (check my post on how to do cost savings with LLM for ideas on what to do). Then it will be worth it to develop your own LLM service (at this point, you should have plenty of money for that).
The goal, when prototyping, is to come to a POC (Proof Of Concept) or MVP (Minimal Viable Product) as soon as possible and you will have plenty to do with just the development of your application. No need to add the creation of an LLM service on top of that.
Conclusion
Let me conclude this blog post in the following way:
- You are just playing by yourself with LLMs —> Don’t hesitate, go for the Local LLM, it will be a blast !
- You are prototyping something and you want to share it / make people pay for it —> Use LLM services (90% of the cases)
- You have millions of LLM api call and very big bills —> Think about developing your own LLM service (check also my post on how to reduce your LLM bills).
Afterward
I hope this post helped you and taught you many things. I will update this post with more nuggets from time to time. Don’t forget to check my other post as I write a lot of cool posts on practical stuff in AI.
Cheers !